Unlocking the Power of Data Annotation: Exploring Annotation Tools for Image Segmentation
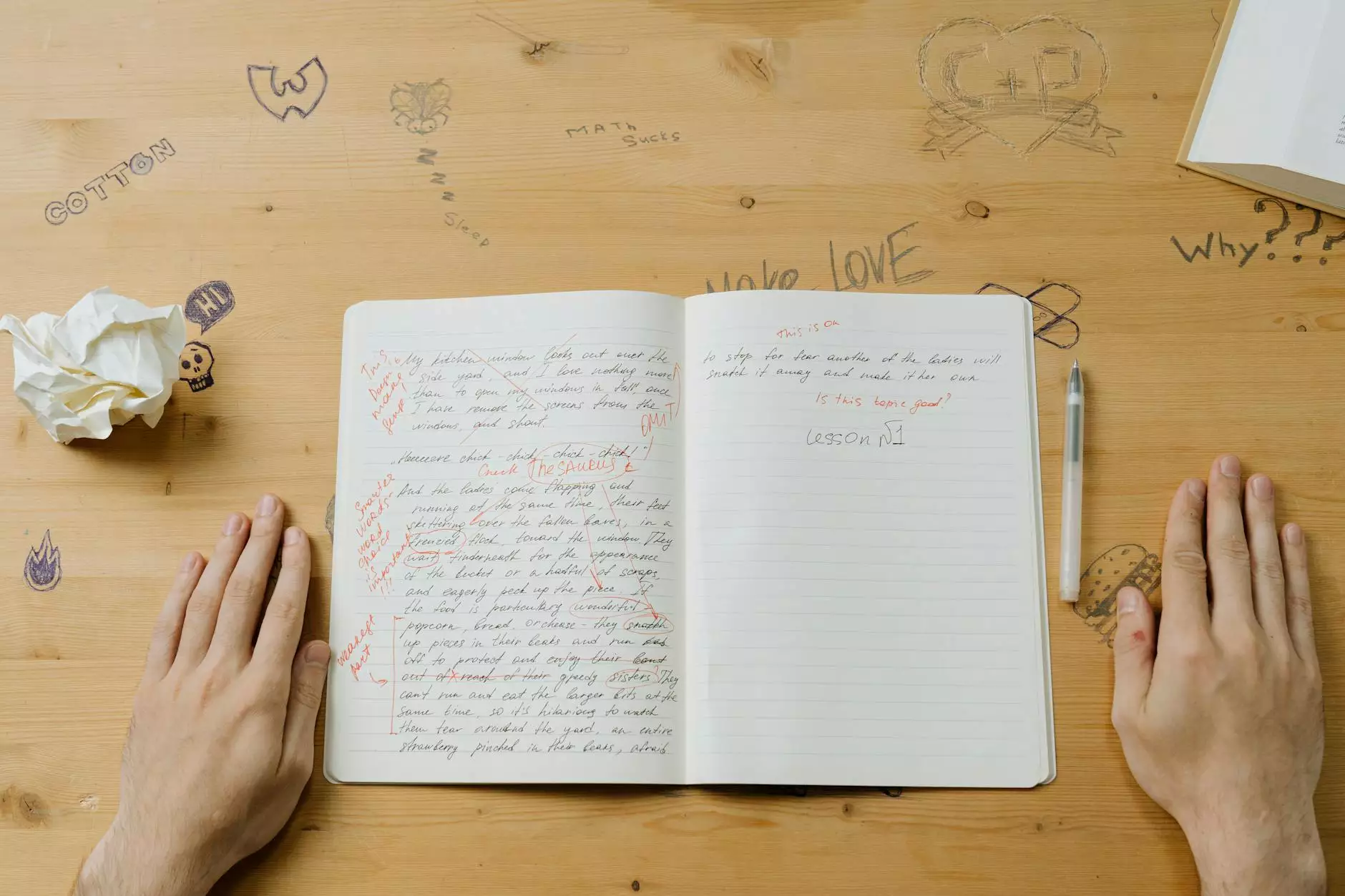
In the age of artificial intelligence (AI) and machine learning (ML), data is more valuable than ever. Companies and organizations depend on high-quality data to train algorithms that drive innovation and efficiency. One crucial aspect of preparing data for machine learning is data annotation, particularly in the context of computer vision. This article delves into the significance of annotation tools for image segmentation, emphasizing how they can transform the way businesses manage and utilize visual data.
The Importance of Data Annotation in Machine Learning
Data annotation involves labeling or tagging data so that machine learning algorithms can understand it. In particular, image segmentation requires precise annotations to identify and classify different segments within an image. This process is vital for a wide range of applications including, but not limited to:
- Autonomous Vehicles: Image segmentation helps identify objects such as pedestrians, vehicles, and road signs.
- Healthcare: Annotations enable the accurate identification of disease markers in medical imaging.
- Retail: Businesses can analyze consumer behavior through object recognition and segmentation in images.
Understanding Annotation Tools for Image Segmentation
Annotation tools are software applications designed to assist in labeling data. When it comes to image segmentation, these tools provide functionalities that streamline the annotation process, yielding faster and more accurate results. Below, we explore the essential features of an ideal annotation tool for image segmentation.
Key Features of Effective Annotation Tools
When selecting a data annotation tool for image segmentation, consider the following key features:
- Intuitive Interface: A user-friendly interface significantly reduces the learning curve and enhances productivity.
- Support for Multiple Annotation Formats: The tool should support various formats, including polygons, bounding boxes, and masks, to accommodate different segmentation requirements.
- Collaboration Tools: A good annotation platform should allow multiple users to collaborate effectively, ensuring consistency and quality in annotations.
- Integration with Machine Learning Pipelines: Seamless integration with ML frameworks is crucial for deploying annotated data into algorithms quickly.
- Quality Control Mechanisms: Features such as review processes and performance analytics help maintain high annotation standards.
Benefits of Using Annotation Tools for Image Segmentation
Employing a robust annotation tool specifically designed for image segmentation offers numerous advantages:
- Increased Accuracy: Manual annotations are prone to errors. Utilizing specialized tools enhances precision and reduces discrepancies.
- Time Efficiency: Automated features and guided workflows expedite the annotation process, saving valuable time.
- Scalability: Businesses can manage extensive datasets with ease, allowing for the rapid advancement of machine learning models.
- Enhanced Collaboration: Teams can share insights and improve the quality of annotations through collaborative features.
- Cost-effectiveness: A streamlined annotation process reduces the costs associated with data preparation, maximizing overall ROI.
The Role of Keylabs.ai in Image Segmentation
Keylabs.ai stands at the forefront of the data annotation industry, offering cutting-edge data annotation tools that cater to a wide range of sectors. With a focus on image segmentation, Keylabs.ai provides innovative solutions designed to enhance data quality and accelerate project timelines.
Why Choose Keylabs.ai?
The following attributes make Keylabs.ai a leading choice for businesses seeking annotation tools:
- AI-Powered Features: Keylabs.ai leverages artificial intelligence to assist human annotators, ensuring high-quality results.
- Customizable Workflows: The platform offers flexibility to accommodate specific project needs, enabling tailored experiences for users.
- Comprehensive Support: Clients receive support throughout the annotation process, including training and ongoing assistance.
- Data Security and Privacy: Keylabs.ai adheres to stringent data protection regulations, ensuring that sensitive data remains secure.
Real-World Applications of Image Segmentation
Understanding how image segmentation is employed across various industries can showcase the tool's versatility:
1. Healthcare and Medical Imaging
In the medical field, accurate image segmentation is crucial for diagnosing and treating patients. Automated systems can identify tumors, measure anatomical structures, and assist in surgical planning with remarkable accuracy. This precision can lead to better patient outcomes and enhanced operational efficiencies.
2. Autonomous Vehicles
Self-driving cars rely heavily on image segmentation to interpret their surroundings. By accurately identifying pedestrians, traffic lights, and obstacles, vehicle systems can make informed decisions, ensuring the safety of passengers and pedestrians alike.
3. Agriculture
In agriculture, segmentation tools can analyze plant health from drone footage or satellite images. By identifying areas requiring attention, farmers can optimize their resources, leading to improved yields and sustainability.
4. Retail and E-commerce
Retailers leverage image segmentation to analyze customer interactions with products. By understanding how consumers engage with visual content, businesses can tailor their marketing strategies and improve customer satisfaction.
5. Security and Surveillance
In security, image segmentation enhances the capabilities of surveillance systems. By accurately detecting intrusions and identifying individuals, these systems improve safety and reduce response times.
Challenges in Image Segmentation
While annotation tools greatly enhance efficiency and accuracy, there are still challenges that businesses must navigate:
1. Data Quality
Ensuring high-quality input images is vital. Poor image quality can lead to inaccurate annotations and affect the performance of machine learning models.
2. Subjectivity in Annotations
Human annotators may interpret images differently, leading to inconsistencies. Clear guidelines and effective training are essential to mitigate this risk.
3. Time Constraints
Depending on the project’s scale, annotation can be time-consuming. Balancing speed without compromising quality is a common challenge.
Future Trends in Data Annotation
The field of data annotation is rapidly evolving. Here are some trends to watch for:
- Increased Automation: As AI technologies advance, automation will play a larger role in the annotation process, further enhancing efficiency.
- Integration with Augmented Reality (AR) and Virtual Reality (VR): The convergence of AR/VR technologies with annotation will unlock new possibilities, especially in training and simulations.
- Focus on Ethical AI: As data privacy concerns grow, ethical considerations related to data usage and annotation practices will come to the forefront.
- Expansion of Annotation Types: The demand for diverse annotation types, such as 3D point cloud segmentation, will increase as industries evolve.
Conclusion
In conclusion, the role of annotation tools for image segmentation is critical in today's data-driven landscape. By choosing effective data annotation tools like those offered by Keylabs.ai, businesses can significantly enhance their data quality and operational efficiency. The future of data annotation is bright, with advancements promising to not only improve accuracy and efficiency but also expand the ways in which businesses can leverage visual data for competitive advantage.
As industries continue to adopt AI and machine learning solutions, the need for high-quality annotated data will remain paramount. Explore the potential of Keylabs.ai today and take the first step toward unlocking the full capabilities of your data.