Empowering Machine Learning: The Definitive Guide to Image Annotation Tools
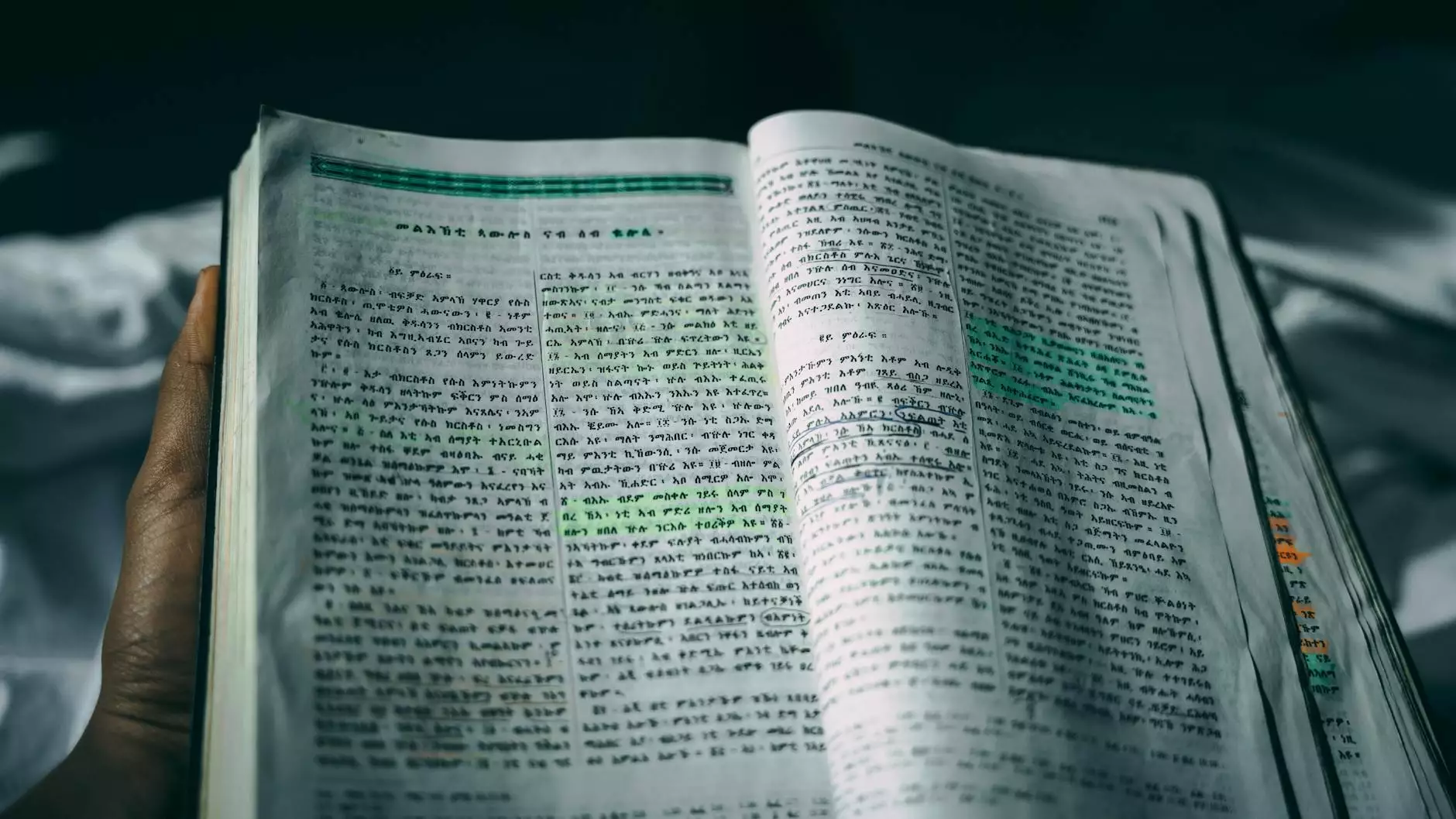
In today's rapidly evolving technological landscape, the role of machine learning has become increasingly pivotal across various sectors. For machine learning algorithms to perform optimally, they require access to vast amounts of accurately labeled data. This is where image annotation tools for machine learning come into play, facilitating the classification and interpretation of visual data. In this comprehensive guide, we will delve deep into the significance of these tools, their functionalities, and how they significantly bolster AI development.
Understanding Image Annotation
Image annotation refers to the process of labeling and tagging images to make them understandable for machine learning models. By providing these models with clearly annotated images, developers can enable machines to recognize and distinguish between various objects and tasks. This process is crucial for training algorithms in several computer vision applications, such as object detection, image segmentation, and facial recognition.
The Importance of Image Annotation in Machine Learning
Effective image annotation serves as the backbone of machine learning, especially in fields that rely heavily on visual data. Here are several reasons why it is vital:
- Improved Accuracy: Well-annotated images lead to better predictive accuracy by reducing misinterpretations by AI models.
- Efficiency in Training: With accurate labels, training times can be significantly reduced, leading to quicker deployment of machine learning models.
- Scalability: Annotation processes can be scaled to accommodate vast datasets, crucial for industries such as autonomous driving and healthcare.
- Data Diversity: By annotating a wide variety of images, you can ensure that your models are trained on a comprehensive and diverse dataset, enhancing their performance in real-world applications.
Exploring Types of Annotation for Image Data
There are various types of image annotation methods employed in machine learning, each tailored for specific applications. Understanding these methods is essential for selecting the right approach for your project.
1. Bounding Box Annotation
This is one of the simplest and most common methods where bounding boxes are drawn around objects of interest in an image. This type of annotation is highly effective for object detection tasks, enabling machines to learn the location and dimensions of the target objects.
2. Semantic Segmentation
Semantic segmentation involves labeling each pixel in an image, categorizing pixels based on the object they belong to. This is crucial for tasks requiring a detailed understanding of the image context, such as in self-driving cars or medical image analysis.
3. Instance Segmentation
Similar to semantic segmentation, instance segmentation involves segmenting each object instance in an image but differentiates between different objects of the same class. This technique is particularly useful in crowded scenes, such as person detection in photos, where distinguishing between individual subjects is necessary.
4. Landmark Annotation
This method involves placing markers on specific points of interest in images, commonly used in facial recognition tasks to identify features such as eyes, nose, and mouth. Landmark annotation plays a vital role in applications such as augmented reality and biometric security systems.
Keylabs.ai: Revolutionizing Your Annotation Process
At Keylabs.ai, we are proud to offer a robust and efficient data annotation platform that empowers businesses in their AI development. Our image annotation tool for machine learning is designed with advanced features that prioritize accuracy, speed, and scalability.
Why Choose Keylabs.ai for Your Image Annotation Needs?
- User-Friendly Interface: Our intuitive platform is designed to facilitate seamless user experiences, allowing both experts and newcomers to work efficiently.
- High-Quality Annotations: Our rigorous quality control processes ensure that all annotations meet the highest standards, providing reliable datasets for training.
- Flexible Solutions: We offer customizable annotation options tailored to specific project requirements, ensuring all needs are met.
- Rapid Turnaround: With our optimized workflows, we deliver high volumes of annotated data in record time, keeping you on track with your project timelines.
- Scalability: Whether you are a startup or an established enterprise, our platform can accommodate your data annotation needs as you grow.
Best Practices for Image Annotation
To achieve the best outcomes from your image annotation efforts, consider the following best practices:
- Clear Guidelines: Establish clear annotation guidelines to maintain consistency across annotations and avoid ambiguity.
- Quality Control: Regularly review the annotated data for quality assurance to avoid errors that could compromise machine learning outcomes.
- Utilize Automation: Leverage automated annotation features where applicable, but always include human oversight for complex tasks.
- Iterative Approach: Continuously refine your annotation processes based on feedback and performance metrics from machine learning models.
Challenges in Image Annotation
While image annotation is essential for developing robust machine learning models, it does come with its set of challenges. Business leaders must be aware of these challenges to develop effective strategies for mitigation.
1. Time-Consuming Processes
Depending on the scale and complexity of the images, annotation can be a lengthy process, particularly when manual efforts are involved.
2. Variability in Quality
Annotation quality may vary significantly based on the annotators’ expertise and understanding of the tasks, which can skew model training.
3. Cost Constraints
High-quality annotation services can be costly, especially for large datasets, posing budgetary challenges for organizations.
4. Adaptability to Different Domains
Not every annotation tool can cater to the specific needs of different industries or use cases, making it essential to choose the right solution.
Future Trends in Image Annotation
The landscape of image annotation is constantly evolving, and staying ahead requires awareness of emerging trends:
1. Increased Automation
With advancements in AI, automation in image annotation is becoming more prevalent, considerably reducing the time required for data preparation.
2. Collaborative Annotation Platforms
Future annotation tools will likely focus on collaboration, allowing teams to work together on projects in real time, enhancing efficiency and accuracy.
3. Integration with Machine Learning Frameworks
Image annotation tools will increasingly integrate more seamlessly with popular machine learning frameworks, streamlining the workflow from annotation to model training.
4. Enhanced Quality Control Mechanisms
Emerging tools will incorporate advanced quality control features to automatically detect and correct annotation errors, ensuring high-quality data.
Conclusion
In conclusion, image annotation tools for machine learning are crucial components that drive the development and success of machine learning applications. By providing accurate, high-quality labeled data, these tools facilitate the training of powerful AI models that can perform complex tasks. With platforms like Keylabs.ai, organizations can harness the full potential of image annotation tools, ensuring they stay competitive in the ever-changing tech landscape. Embracing advanced data annotation solutions is not merely an option; it is a necessity for businesses aspiring to lead in the domain of artificial intelligence.
Investing in an efficient image annotation tool today can open doors to unprecedented opportunities and innovations in AI and machine learning for tomorrow. Dive into the world of image annotation with Keylabs.ai and transform your data into actionable insights!